Today’s world is inundated with data. Everything from cloud-based software applications to the DNA in your body contains useful information – data. Data is the most powerful tool empowering organizations across a spectrum of industries to establish patterns and correlations between seemingly random events.
According to Gartner, the rate of data growth globally in 2011 was around 59%. Business enterprises are among the top users. They use data to predict customer expectations, do effective product development and make informed business decisions.
Here are some of the major brands that leverage data to achieve key business outcomes.
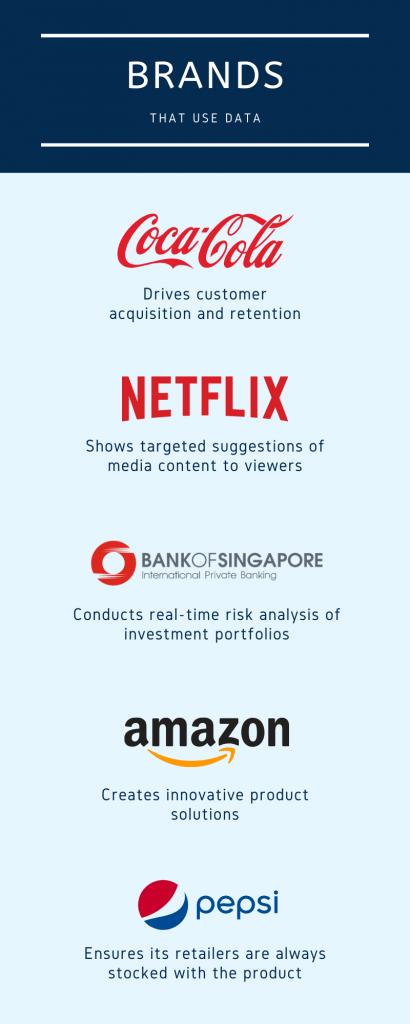
However, take note that even the slightest inaccuracy in data can cause far-reaching damage across the organization. According to Gartner, poor data quality can lead to an average annual loss of $15 million for businesses. This makes Data Quality Management (DQM) a top priority for your organization.
What is Data Quality Management?
Data Quality Management refers to a set of practices that ensure your business is maintaining high-quality data. It incorporates everything from the acquisition of data to its processing, analyses, and distribution across the organization. The goal is to ensure that powered by high-quality data, companies make informed decisions that lead to profitable outcomes.
Why does your business need a dedicated Data Quality Management strategy?
Bad data can not only hinder your processes but also cost you a lot of money. A study by RingLead revealed that bad data costs $100 per record. Given a 100,000-record database, it can lead to a hefty cost of $3.9 million over 3 years.
The cost per record resulting from bad data can be attributed to the following reasons:
- Printing and emailing to wrong addresses
- Duplicate records taking up extra server space
- Redundant workflows for duplicate records
- Incorrect marketing segmentation
- Sales disputes over the same leads
- Losing dissatisfied customers
You can avoid this cost by implementing a dedicated data management strategy for your business.
Here’s how it can benefit your company:
- Your business processes become more efficient because you get accurate data every time.
- You get a complete and accurate view of your business with actionable information on customers, competitors, and vendors.
- You can make more informed decisions and be confident about achieving the desired business outcomes.
5 steps to implementing Data Quality Management in your business
Now that you understand the importance of Data Quality Management, let’s discuss ways to implement it within your organization.
1. Set up an organizational structure
Any business process needs people to carry out and monitor it. Create a dedicated team for Data Quality Management and assign them roles based on their skills and qualifications.
Some key people in your DQM team include:
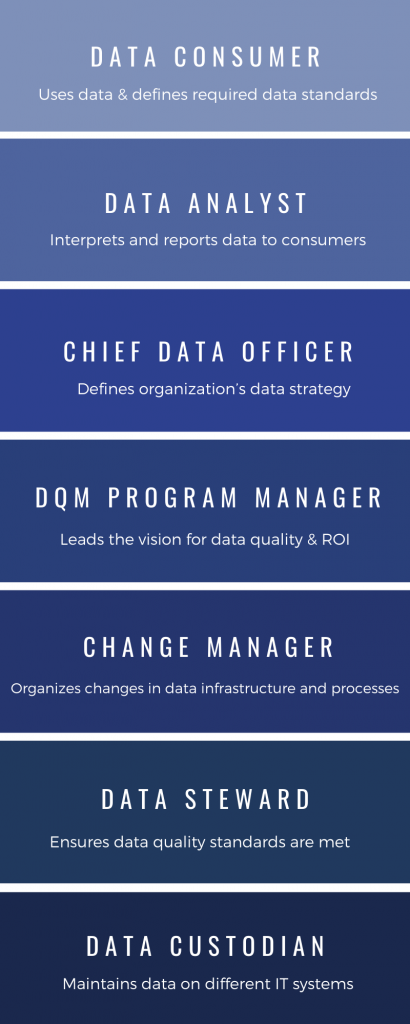
2. Define data quality
You don’t really know whether you’re meeting data quality standards unless you define what you mean by quality data.
Here are 7 key parameters:
- Accurate: Is the data accurate?
- Valid: Does it conform to the values of the given data set?
- Complete: How much of the relevant data has been collected?
- Consistent: Is there a consistent method to collect and store data for different data sets?
- Timely: Is the data up-to-date and relevant to the current circumstances?
- Unique: What is the level of detail or granularity at which data is collected?
- Accessible: How easy is it to access or collect the data?
Different industries may have varying data needs and can choose to define data quality accordingly.
3. Initiate data profiling
Once you have defined these parameters, you can conduct actual Data Quality Management. This primarily involves data profiling – an audit process that investigates and rectifies data quality issues. These issues may include duplication, or lack of consistency, accuracy, and completeness.
Data warehousing guru, Ralph Kimball, came up with a data profiling process comprised of four steps:
- Test data quality at the start of every project to see if it’s appropriate for analysis.
- Diagnose and fix quality issues in the source of data, before you move data to the target database.
- Detect any remaining data quality issues while moving data from source to the target using the Extract-Transform-Load (ETL) method.
- Identify key interrelationships and unforeseen business rules that may affect the data profiling process, and refine the profiling methods as needed.
There are many open-source tools in the market you can use to automate data profiling, as well as a range of paid data profiling tools for larger-scale enterprise use-cases.
Although most companies opt for traditional data profiling done by data engineers, it is time-consuming and cumbersome. At a time when data is pouring in from multiple sources and data profiling is more crucial than ever, it makes more sense to use AI-driven data warehouses that can automate data profiling.
4. Report and monitor data
DQM reporting is the process of monitoring, reporting and recording exceptions in data and in the business processes that depend on data. You can capture these exceptions using business intelligence (BI) software. It helps identify the location of data exceptions.
Your data specialist team can now aggregate these exceptions to create patterns that determine why your data collection and processing deviates from the set standards. Now that you have identified the problem, you can strategize your data remediation process.
5. Repair data
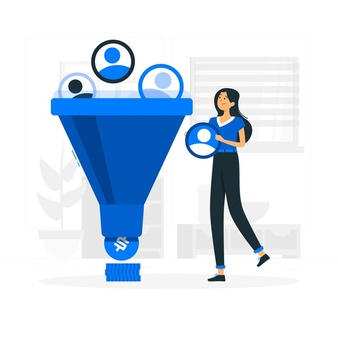
Data remediation involves determining the best way to repair data and to implement the change in data collection, processing, and analysis methods.
You can use the data profiling tools listed above to clean your company’s data. Next, comes the task of re-initiating the processes that depend on defective data. This may include re-running some reports, campaigns or financial documentation with the now clean data.
This is also the step where you should review and improve data quality rules. Once you have refined data quality standards and have high-quality data, your critical business processes will run efficiently.
KPIs for measuring the success of your DQM strategy
You can track the following KPIs to see if your DQM strategy is successful or not.
- The ratio of errors relative to the entire data set
- The number of empty values
- Data insights time-to-value realization
- Data transformation error rate
- Data storage costs per unit of data
Data Quality Management is a continuous process
Data Quality Management is not a one-time sprint. You remediate your data and business processes once. Great! Don’t stop here. A good DQM strategy is continuous and geared for constant improvement.
Given today’s data volumes, carrying out Data Quality Management manually can be grueling. You can use automated Data Quality Management software solutions to implement a more iterative and continuous process for honing your data and processes.
I do agree that having data quality monitoring services can really make processes more efficient as accurate data is processed and produced. If I do have a business that deals with a lot of data, of course, I would only understand why this integration in technological innovation services is important. Not only that you ensure proper operations, you are also at peace with quality data interpretation.